-
Apply OSSEs to 1) quantify the utility of future observing systems (Surface Water Ocean Topography (SWOT) altimetry, ROCIS, UGOS-3 collaborators’ observations) and 2) determine optimal and rapid adaptive sampling strategies.
-
B. Cornuelle, A. Moore, R. He, J. Sheinbaum, G. Gopalakrishnan, S. Bulusu, D. Dukhovskoy, E. Chassignet, and S. Morey in collaboration with NRL and NOAA
Objectives
Building upon prior activities conducted by project team members during UGOS-1, OSSEs will be applied to quantify the utility of future observing systems and guide optimal and rapid adaptive sampling strategies.
To balance the need to increase observational capabilities in the Gulf of Mexico (GoM) with constraints of available resources, we must evaluate the impact of current and proposed observations on model forecast skill. This can be accomplished using OSSEs, which compute ocean analyses using baseline observations synthetically sampled from a model “nature run” (NR) that is taken to be the true ocean. The observations are analyzed and the resulting ocean estimates, including forecasts, are compared to the NR, computing errors for critical quantities of interest (QOI) such as the LC front position, currents, or LC eddies. The analysis is repeated with other candidate sets of observations and recomputing the estimates and skills. By comparing ensembles of analyses and ensuing forecasts, the impact of the data withheld/added can be quantified. OSSEs provide understanding of which measurements are critical and how to sample them to further improve GoM analyses and predictions (i.e., adaptive sampling).
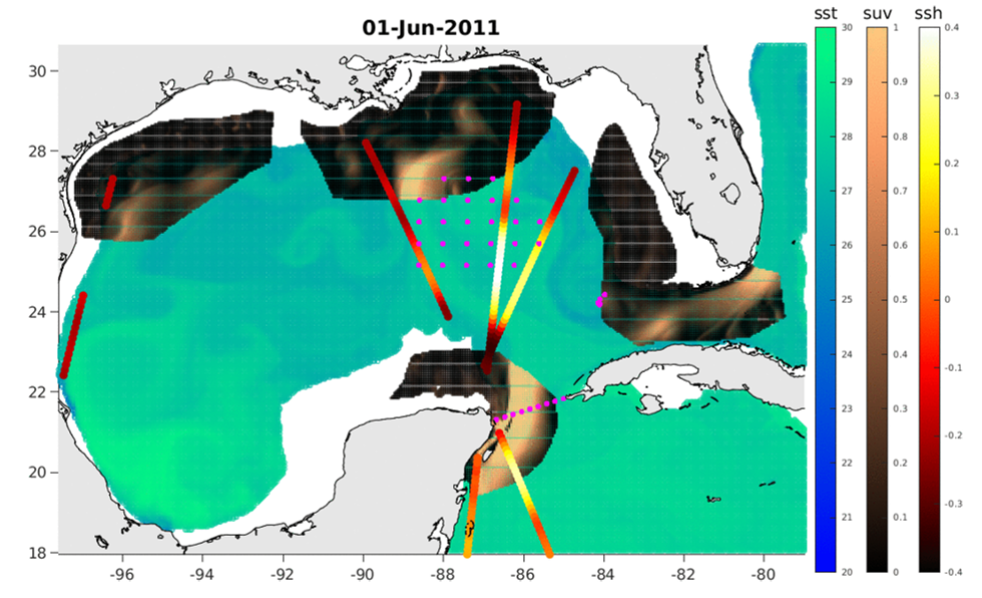
As part of UGOS-1, members of this proposal team performed OSSEs differentiating observations into surface and sub-surface layers and included ocean observations from in situ measurements, such as PIES (Pressure Inverted Echo Sounders), High Frequency (HF) Radar, and deep moorings, as well as satellite-derived sea surface height (SSH) and temperature (SST), to identify what type of observations and which regions produce the most skillful GoM forecasts with lead times of 1 to 3 months. This work considered two LC scenarios: an energetic LC state with multiple LC eddy (LCE) detachment and reattachments, and a less-energetic LC state with LC growth.
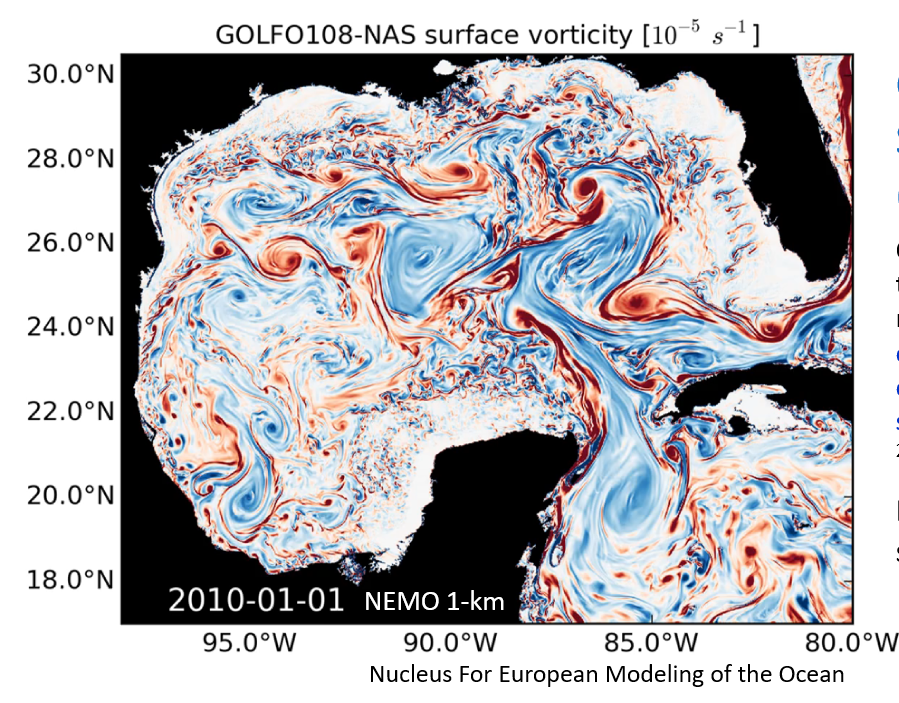
The results show that even a few observations of the vertical structure at strategic locations can lead to significant forecast improvement. Particularly challenging for the forecast models are the timings and fate (either reattachment or separation) of LCE detachments. Results from OSSEs and previous observational efforts (e.g., Donohue et al, 2016) indicate that model representation of LC meanders and instabilities is important for LCE shedding prediction. We find that adding observations to those that are typically assimilated (i.e., SSH, SST, sparse profiles) is required to properly constrain the key LC features that impact LCE shedding processes. Routine monitoring of 3-D temperature, salinity, and velocity structure for the entire GoM is not tractable. Thus, strategic use of observational assets through adaptive sampling can provide the information needed to improve model forecasts at crucial times, yet with manageable costs.
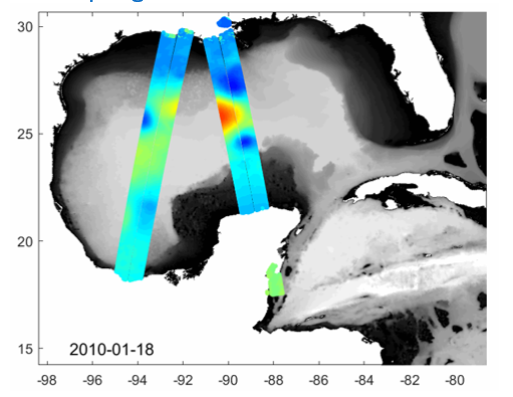
Methodology and Implementation
We will conduct OSSEs for selected LC states to understand the impact of observations on the LC forecasts. The main purpose is to examine the LC and LCE predictability for a period of one to three months for representative LC state realizations, with a focus on extended LC states.
As part of the UGOS-1 project research performed by members of this proposal team, OSSEs were conducted using CICESE’s GoM configuration of the Nucleus for European Modelling of the Ocean (NEMO) (Jouanno et al., 2016, 2018) 1 km solution as a NR. We will expand upon the OSSE capabilities provided by this NR by enhancing it and complementing it with an additional NR, a recently completed HYCOM GoM 1 km free-running simulation. We will use 1) SWOT altimetry by sampling the NR using the SWOT observation simulator; 2) in situ synthetic observations by sampling the NR for near-surface currents (e.g., HF Radar, drifters, and ROCIS) and temperature profiles (e.g., from AXBTs) for the period 2014-2018 when the LC exhibited anomalous behavior. We will also include observations (e.g., moorings, PIES, RAFOS, surface drifters drogued at various depths, and gliders) from collaborating UGOS-3 consortia.
We will evaluate the impact of data types on the predictability of LC and GoM features and fields and suggest adaptive sampling strategies to enhance the LC and LCE prediction (i.e., the Grand Adaptive Sampling Experiment – see Task 1.5 and Section 8). The OSSE estimates will be compared with NR solutions for SSH, SST, temperature at 200 m depth and deep eddy kinetic energy (EKE) to assess the impact of the observations using skill metrics such as root mean square difference (RMSD) for fields and Modified-Hausdorff-Distance (MHD) for contours like the LC front. We will also explore novel metrics that can be used to clearly define the LC frontal features and quantify key aspects of LC evolution.
The CICESE team will make several improvements to their NR simulation, motivated by the UGOS-3 objectives. First, the number of vertical levels will be increased from 75 to at least 150 and possibly 300 levels, depending on computer resources. This will provide a better representation of high vertical mode dynamics, intra-thermocline eddies, the deep penetration of the near-inertial energy injected by winter cold surges and tropical cyclones, and deep circulation and its interaction with topography. Tidal forcing will be added since internal tide energy fluxes in the GoM affect in situ measurements and deep-water mass transformations. Finally, hourly atmospheric and surface wave fields from the ERA5 reanalysis will be used to force the model.
SIO’s MITgcm and NCSU and UCSC’s ROMS modelers will augment the OSSEs with array design calculations that identify regions that strongly control LC evolution. Computing quantitative indices for LC evolution (e.g., frontal location) is still application-dependent (e.g., frontal location with respect to an operational region) and somewhat of a research question. In addition to the OSSEs, we will conduct ensemble and adjoint sensitivity experiments to identify key regions that control important aspects of the LC at increasing lag times. For example, LCFEs are thought to control LCE separation in some instances. We have traced back the sensitivity of a measure of LCFE strength to ocean model state at earlier times and found regions of control on the continental shelf in the western GoM, in the Yucatan Strait, at Cozumel Island, and in some parts of the deep GoM (Gopalakrishnan et al., 2013). These same tools are useful for adaptive sampling, i.e., optimization of the deployment of mobile observation platforms.
Progress
Updates coming soon.